Risk-Scoring For Journal Entries Using Data Analytics
Try Now & See it For Yourself.
Great reliance is placed on timely and accurate financial statements
Journal entries posted to the general ledger are the source of balance sheets, income statements, and statements of cash flows. To mitigate the risk of inaccurate journal entries, deploy data analytics for scoring each journal entry posting. Risks can arise from a variety of factors such as weak internal controls, aggressive fraudsters, and the increasing complexity of financial reporting systems.
What Are Journal Entries?
Journal entries are simply the debit and credit transactions that are applied to general ledger accounts. These entries are, in most organizations, automatically posted by one or more ERPs. Manual journal entries can also be posted by parties with the appropriate duties. The key fields that occur in journal entries are the amount, the GL account, the posting date, the DR/CR indicator, reference numbers, cost center, the user, and the creation date.
The Impact of Fraud
The ACFE conducts an annual survey of occupational fraud schemes. Although financial statement frauds are relatively low in number, the average financial statement fraud is nearly one million dollars, quite a bit more than the other categories.
ACFE "Report to the Nations: Global Study on Fraud and Abuse 2020"
Risk Scoring
Risk scoring is an effective way to isolate the journal entries that may indicate fraud, weak controls, and errors.
Here are the steps you can take to build a risk-scoring algorithm using your favorite data analysis tool.
- Review risk indicators with your risk management Department and with your accounting department. This will ensure a common, unified approach in the assessment of risks.
- Include characteristics that are common to industry, location, etc…as well as those that may be unique to your organization.
- Apply risk scores to each transaction based on the transaction's characteristics.
- Sum the risk scores.
- Start your review with highest-scoring transactions.
- Score can be a simple binary score (1 or 0).
- Alternatively, you can weight each characteristic's score for impact and risk.
In this example, we've created four tests and applied a binary risk score if the journal entry meets the test. Each journal entry's score is then summed for an overall risk score. The highest-scoring entries should be reviewed first, followed by the next-highest, and so on. You can run several analytics on the high-scoring items, such as aggregating them by the person making the entry, the time period, the and the GL account. You can create a binary score using your data analysis application's conditional computed field functionality.
figure2: Risk-Scoring example
Basic Tests
- Outliers by account
- Infrequent users
- Round amounts
- Unauthorized users
- Manual entries
- Immediately prior to or after end of period
- Amounts just below approval limits
- Holidays
- Weekends
- Keywords
- Duplicates
- Seldom-used accounts
- Large credits to top-line revenue
- Large credits to other revenue accounts
- Whole-Population outliers
-
Test 01: Holidays
Even though some automated postings can be generated on holidays, it's worth examining such instances to ensure that you're aware of them and their impact.
In Arbutus Analyzer, you can create a file of holidays, join the journal entry file to the holiday file using the dates as the key fields, and assign a value of 1 if the entry was posted on a holiday. -
Test 02: Weekends
The second test is to review weekend postings. This is straightforward in many applications where you can build a conditional computed field using the Weekend function to test the dates. Weekends and holidays are of particular significance if you're reviewing manual journal entries.
-
Test 03: Keywords
Keyword searches of comment or description fields are the third test. You can create a keyword list in Notepad, including not only standard keywords but also those that are particular to your organization and your industry. A conditional computed field using the ListFind function will identify postings where any keyword is detected in the text fields. The condition expression assuming "JE Keywords.txt" is the keyword file and the field to be searched is the Comment field, will look like this:
ListFind("JE Keywords.txt",Comment) -
Test 04: Duplicates
Duplicates or fuzzy duplicates are popular tests. Looking for the same user, same account, same debit/credit indicator, same amount within 14 days of each other will exclude bi-weekly recurring transactions. Reducing false positives is a good way to make the reviews more efficient. You can run the Duplicates command with the appropriate parameters to send the results to a new file. You then join that file back to the master file and create a binary score of 1 if the journal entry appears in both lists.
-
Test 05: Seldom-Used AccountsSeldom-used accounts are often used by fraudsters in an attempt to keep their work hidden. Since it's rare to expect transactions in those accounts, it would be worthwhile to include such postings in your review.
- Classify on the GL account field to a new file.
- In the new file, run the Statistics and Stratify commands on the Count field to determine what your threshold, in terms of the number of transactions, should be.
- Extract the records filtering on the Count field to isolate only the low-number accounts.
- In the JE file, create an All-Primary Join to the file created in step 3, using the account numbers as the key fields.
- Create a conditional computed field to risk-score the journal entries.
-
Test 06: Large Credits to Top-Line Revenue Accounts
There shouldn't be any postings to top-line revenue accounts. To identify them, use the top-line revenue account number as the key field in the formula. Assuming the top-line revenue account number is "0000400000" and your materiality threshold is 2 standard deviations above the average, your condition expression would be:
GL_Account = "0000400000" AND Amount_in_local_currency > average + 2 standard deviations -
Test 07: Large Credits to Other Revenue Accounts
Similarly, we can also look at large credits to other revenue accounts. Assuming all income statement revenue accounts begin with "00004", your condition expression would filter for those accounts and would exclude the top-line revenue account:
GL_Account = "00004" AND GL_Account <> "0000400000" AND Amount_in_local_currency > average + 2 standard deviations
-
Test 08: Whole-Population Outliers
This test involves identifying postings where the amounts are more than 2 standard deviations above the population average. We can run the Statistics command to generate the average and the standard deviation. The next step is to build a conditional computed field that scores the posting if it is above our threshold.
The condition expression would be:
Amount_in_local_Currency > 4634.26 + (2 * 26570.21) -
Test 09: Outliers by AccountYour outliers testing can be more granular if you make your populations based on the account. This will consider the size of the typical transaction for each account rather than relying on statistics based on the entire population.
You can calculate the average and standard deviation for each account, then apply the risk score appropriately:
- Run the Summarize command by account to a new file including the mean and standard deviation for the amount field. This can be done by selecting the amount field twice and changing the Type values to "AVG" and "STDDEV". The output will contain one row per account with the mean and the standard deviation for each account.
- In the new file, calculate the 2 standard deviations threshold for each account.
- Join to JE file based on account number and include threshold amount.
- Risk score = 1 if amount is in excess of the threshold for the account.
Summarize: Click "Choose" in the "Fields to process" section. -
Test 10: Infrequent Users
You can use the same process that were used in identifying seldom-used accounts.
-
Test 11: Round Amounts
Round amounts are not common for naturally-occurring transactions and thus may be higher-risk than other amounts. There are many cases of fraudsters using round amounts in their schemes, sometimes repeatedly.
You might want to establish a materiality threshold so that you would be considering the size of the posting. Let's use $10,000 currency units as our threshold when we build our condition using the Modulus function. This function calculates the remainder after the amount is divided by the $10,000 threshold:
MOD(Amount_in_local_currency,10000) = 0
-
Test 12: Unauthorized Users
Unauthorized users are rare, but they've been known to circumvent controls in order to post entries. Obtain a file of authorized users and join it to the transaction file based on the user field. Any unmatched records will indicate that the user was not in the authorized users file.
-
Test 13: Manual Entries
Manual journal entries can sometimes override system controls, and they're not automatically generated, so it's a good idea to review them as well. Identify the field and the value(s) that indicate a manual entry and create the condition expression accordingly. In our example, the field is "Document_Type" and the two indicators of manual entries are the codes KG and ZH:
MATCH(Document_Type,"KG","ZH")
-
Test 14: Postings Around the Period End
Postings around the end of the period are also suspect. Many organizations have been known to post amounts to sales in the waning days of a period, and then reverse those transactions immediately after the beginning of the next period. This inflates sales figures and bonuses, and it paints a rosier picture of the firm's financial state. The impact is also felt in the stock price.
If we establish the five days before and five days after the period end of March 31, 2020 to be relevant, our condition expression would look like this:
BETWEEN(ABS(Posting_Date - `20200331`),0,5)
-
Test 15: Amounts Just Below Limits
As auditors, we're all familiar with the old scheme whereby someone doesn't want to bother their direct manager for amounts above their limits. They take it upon themselves to split up the large transactions into two transactions, for instance, each of which is below the individual's approval limit. The test can either be a fixed dollar amount or a percentage below their limits.
- Obtain a file of user limits.
- Join the transactions to this file based on the user as the key field. This will place each user's limit adjacent to each journal entry.
- Create the scoring field using the following condition for transactions within $100 below each user's limit:
BETWEEN(Limit_in_Local_Currency - Amount_in_local_currency,.01, 100)
Summing the Scores
Once all the tests have been created, create another field to sum the scores for each posting. You can then run additional analytics to identify possible trends or commonalities. For example, you may want to use the Stratify command to see the frequency distribution of the total scores.
In this example, we can see that two journal entries had the highest score of 6. Their total materiality is nearly $89,000 or .19% of the total population materiality.
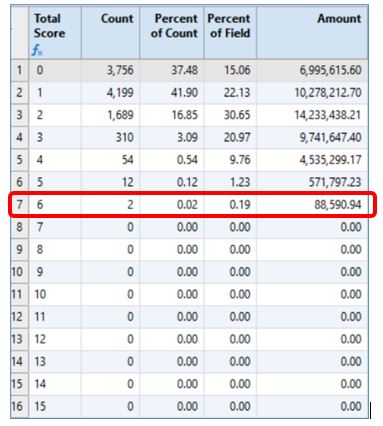
Other analytics to consider are frequency distributions by user, GL account, and other characteristics. The Classify command can produce the user distribution with the scores in descending order. This can be done by selecting the "By Size" and "All items" parameters in the Classify command dialog:
Best Practices
Here are some best practices to keep in mind:
- Discuss risks with the risk management and accounting departments.
- Identify risks that may be particular to your organization, industry, location, etc…
- Review risk scoring algorithms regularly.
- Create a script to automatically execute creation of scores.